Insights
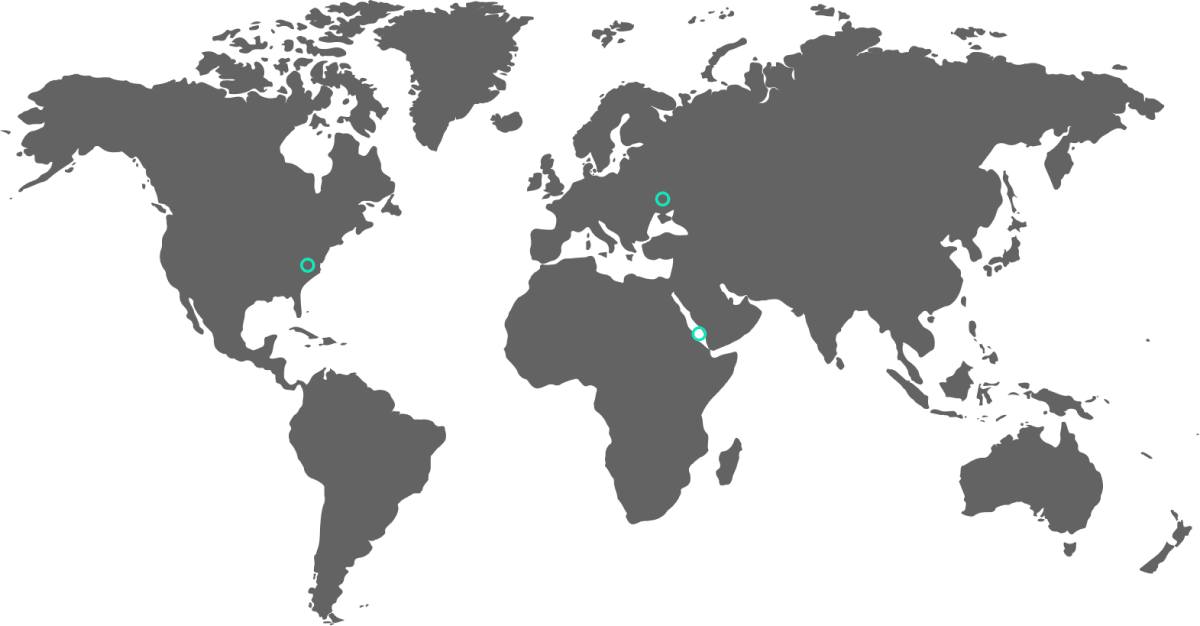
ARLINGTON, VIRGINIA
It took less than an hour for fabricated images of an explosion at the Pentagon to appear on 4Chan, Twitter, Reddit, and other channels on May 22, accumulating 4K mentions before being fact checked by mainstream media.
THE RED SEA
As Houthi-led attacks against vessels transiting the Red Sea have increased, Zignal detected a correlating information operation resulting in an increase of pro-Houthi slogans within the global media environment.
KAKHOVKA, UKRAINE
Within 48 minutes of sunrise on June 6, Zignal’s AI algorithm and critical infrastructure geofence of the Ukraine conflict zone automatically detected damage to the Kakhovka dam.
Leverage Zignal to Understand Key Variables Within the Operational Environment
Political
Global policy, diplomacy, and international elections
Military
Disruptive events, global security, and areas of conflict
Economic
Financial markets, stock manipulation, and supply chain
Social
Beliefs, values, customs, and behaviors within society
Information
Influence operations, technology, and emerging platforms
Infrastructure
Transportation networks, cybersecurity, and facility protection
Physical Environment
Natural disasters, resource supplies, and climate change